New Microsoft Research


Microsoft Research has been at the forefront of developing novel solutions to meet the growing needs of both the technological landscape and the search engine industry. Generative Retrieval for Ranking Answers is one such development; it is a conversational question answering model with game-changing implications for web search. This article delves into Microsoft’s ground-breaking research and what it could mean for the future of search.
An Overview of Rank-Based Answer Generation
Generative Retrieval for Ranking Answers (GCoQA) is a new method for answering questions in natural language conversations developed by Microsoft Research. This model employs generative language models and cutting-edge retrieval methods to enhance the precision and speed with which questions are answered. GCoQA predicts the next word or phrase and ranks passages based on relevance to the given question using autoregressive models and identifier strings.
The Process of GCoQA
To create the identifier strings that stand in for sections of text in a document, GCoQA uses autoregressive models. Titles of pages and sections help readers understand the structure of a document and locate the information they seek. By encoding this data, GCoQA is able to retrieve the appropriate passages and generate answers by employing a different autoregressive model. Using a hierarchical search strategy improves both context comprehension and the quality of the results returned.
Benefits of Using GCoQA
When compared to conventional approaches, GCoQA has many merits. It is more effective in practice because it makes much less use of memory resources. When compared to competing models, GCoQA is both faster and more accurate. The beam search and multiple identifiers that GCoQA generates allow it to rank passages according to how likely they are to contain the correct answer. GCoQA’s performance is enhanced as a result of the fine-grained cross-interaction that allows it to pay closer attention to the conversation context.
Constraints and Difficulties
GCoQA has tremendous potential, but it also has some restrictions and difficulties that must be worked out. Because of its reliance on the beam search technique, GCoQA has trouble remembering expansive passages, and the model’s performance degrades as the beam size gets larger. As a result, ambiguous questions or webpages without meaningful section headings can be a problem for GCoQA because of its reliance on the semantic relationship between questions and passage identifiers.
Implications and Potential Future Uses
While there are still some barriers to entry, GCoQA has the potential to revolutionize search in the future. In more general web search scenarios where identifier strings may not be readily available, researchers at Microsoft are actively exploring the use of generative retrieval. To further investigate their interconnected nature, researchers are interested in combining passage retrieval and answer prediction into a single generative model. These developments may one day allow for more precise and time-saving search results for internet users.
See first source: Search Engine Journal
FAQ
1. What is Generative Retrieval for Ranking Answers (GCoQA)?
GCoQA, or Generative Retrieval for Ranking Answers, is a conversational question answering model developed by Microsoft Research. It uses generative language models and advanced retrieval methods to improve the precision and speed of answering questions in natural language conversations.
2. How does GCoQA work?
GCoQA employs autoregressive models to create identifier strings that represent sections of text in a document. It then uses these identifier strings to retrieve relevant passages and generate answers. A hierarchical search strategy is used to enhance context comprehension and the quality of results.
3. What are the benefits of using GCoQA compared to conventional approaches?
GCoQA offers several advantages over traditional methods. It is more memory-efficient, faster, and more accurate. The model uses beam search and multiple identifiers to rank passages based on their likelihood of containing the correct answer, improving its performance.
4. What constraints and difficulties does GCoQA face?
GCoQA has limitations related to its reliance on the beam search technique. It struggles to remember extensive passages, and its performance degrades with larger beam sizes. Additionally, ambiguous questions and webpages without meaningful section headings can pose challenges for GCoQA.
5. What are the potential implications and future uses of GCoQA?
GCoQA has the potential to revolutionize web search. Microsoft researchers are actively exploring its application in more general web search scenarios where identifier strings may not be readily available. They are also working on combining passage retrieval and answer prediction into a single generative model, which could lead to more precise and time-saving search results for internet users in the future.
Featured Image Credit: Matthew Manuel; Unsplash – Thank you!
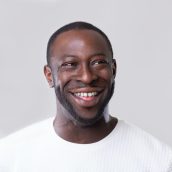
Colin Hughes, a passionate wordsmith and digital raconteur. He ghostwrites for numerous websites that include travel, culture, and lifestyle content. When not traveling for work, he loves to spend his time at home with his husband and two border collies, Reggie and Tuesday.