Tree Of Thoughts Prompting: Unlocking the Potential of Generative AI


In the realm of artificial intelligence (AI), researchers are constantly pushing the boundaries to improve the capabilities of language models. One such advancement is the development of the Tree of Thoughts (ToT) prompting strategy, a method that takes generative AI to new heights by unlocking more sophisticated reasoning methods and producing better outputs. In this article, we will delve into the concept of ToT prompting, its comparison with other strategies, its inspiration from human cognition, and its potential applications.
Understanding ToT Prompting
The ToT prompting strategy was developed by researchers from Google DeepMind and Princeton University as an enhanced approach to guide language models in generating coherent and connected responses. Unlike traditional prompting methods, ToT prompts the language model to follow a tree structure of reasoning steps, allowing for evaluation and selection of viable paths towards finding a solution or completing a task.
ToT prompting goes beyond linear thinking and introduces a more deliberative and conscious approach, similar to the slow, deliberate, and logical decision-making processes observed in humans. By evaluating each step of the reasoning process, the language model can determine the viability of a particular path and make informed decisions about whether to continue or explore alternative branches.
Comparing ToT Against Other Prompting Strategies
To gauge the effectiveness of ToT prompting, the researchers compared it against three other prompting strategies: Input-Output (IO) Prompting, Chain of Thought (CoT) Prompting, and Self-consistency with CoT.
- IO Prompting: This strategy involves providing the language model with a problem to solve and receiving the answer as the output. For example, in text summarization, the input prompt would be to summarize a given article, and the output prompt would be the resulting summary.
- CoT Prompting: CoT Prompting guides the language model to generate coherent responses by following a logical sequence of thoughts. It provides intermediate reasoning steps to solve problems, as demonstrated in the example of calculating the number of tennis balls Roger has after purchasing additional cans.
- Self-consistency with CoT: This prompting strategy prompts the language model multiple times and selects the most commonly arrived at answer. By sampling diverse sets of reasoning paths, it leverages the intuition that complex problems often have multiple correct answers arrived at through different paths.
Drawing Inspiration from Dual Process Models in Human Cognition
The ToT prompting strategy draws inspiration from dual process models in human cognition, which propose that humans engage in two distinct decision-making processes: one intuitive and fast, and the other deliberate and slower.
The “System 1” mode of human cognition involves fast, automatic, and unconscious thinking based on intuition. On the other hand, the “System 2” mode is characterized by slow, deliberate, and conscious thinking, involving careful analysis and step-by-step reasoning before arriving at a decision.
ToT prompting embodies the characteristics of the “System 2” cognitive model by encouraging the language model to follow a series of steps while also evaluating the viability of each step. This approach allows for a more thorough exploration of possible paths and reflects the kind of heuristic-guided search observed in human problem-solving.
The Structure of ToT Prompting
ToT prompting introduces a tree and branch framework for the reasoning process, enabling the language model to explore multiple paths towards finding a solution. Each step of the reasoning process is represented by a “thought” within the tree structure. The language model evaluates each thought and determines whether it is a viable step towards the final solution. If a thought is deemed ineffective, the model abandons that branch and continues exploring other branches until it reaches the desired result.
In contrast, CoT prompting follows a more linear path, instructing the language model to adhere to a predetermined sequence of steps. While CoT is effective in guiding the language model through intermediate reasoning steps, ToT takes it a step further by providing an evaluator step that reviews each reasoning step’s viability.
Illustrations of Prompting Strategies
To provide visual representations of the various prompting strategies, the research paper published schematic illustrations for each approach. The ToT prompting strategy is depicted with rectangular boxes representing individual thoughts within the reasoning process, forming a branching structure. On the other hand, the CoT prompting illustration shows a more linear thought process.
These illustrations highlight the key shortcomings of traditional approaches that use language models to solve problems. Existing approaches often neglect to explore different continuations within a thought process (branches of the tree) and fail to incorporate planning, lookahead, or backtracking to evaluate different options—an essential aspect of human problem-solving.
Testing ToT Prompting with a Mathematical Game
The researchers conducted tests to evaluate the effectiveness of the ToT prompting strategy, using the mathematical card game “Game of 24.” In this game, players utilize four numbers from a set of cards, combining them using basic arithmetic operations to achieve a result of 24.
The results of the tests indicated that the ToT prompting strategy consistently outperformed the other approaches. However, the researchers also noted that ToT prompting might not be necessary for tasks that language models like GPT-4 already handle well.
See first source: Search Engine Journal
FAQ
What is the Tree of Thoughts (ToT) prompting strategy in AI?
The Tree of Thoughts (ToT) prompting strategy is an approach developed by researchers to guide language models in generating coherent and connected responses. It involves following a tree structure of reasoning steps, allowing for evaluation and selection of viable paths towards finding solutions or completing tasks.
How does ToT prompting differ from traditional prompting methods?
ToT prompting differs from traditional methods by introducing a more deliberative and conscious approach, akin to human decision-making. It encourages the language model to evaluate each step of the reasoning process and make informed decisions about whether to continue along a path or explore alternative branches.
What other prompting strategies were compared to ToT prompting in the research?
The research compared ToT prompting against three other strategies: Input-Output (IO) Prompting, Chain of Thought (CoT) Prompting, and Self-consistency with CoT.
What is IO Prompting, and how does it work?
IO Prompting involves providing the language model with a problem to solve and receiving the answer as the output. For example, in text summarization, the input prompt would be to summarize an article, and the output prompt would be the resulting summary.
What is CoT Prompting, and how does it guide language models?
CoT Prompting guides language models to generate coherent responses by following a logical sequence of thoughts. It provides intermediate reasoning steps to solve problems, such as calculating the number of tennis balls someone has after purchasing additional cans.
What is Self-consistency with CoT, and how does it differ from other strategies?
Self-consistency with CoT prompts the language model multiple times and selects the most commonly arrived at answer. It leverages the idea that complex problems often have multiple correct answers arrived at through different reasoning paths.
What is the inspiration behind the ToT prompting strategy?
ToT prompting draws inspiration from dual process models in human cognition, which propose two distinct decision-making processes: intuitive and fast (System 1) and deliberate and slow (System 2). ToT embodies the characteristics of System 2 cognition by encouraging thorough exploration of possible paths in problem-solving.
How does ToT prompting structure the reasoning process?
ToT prompting introduces a tree and branch framework for reasoning, allowing the language model to explore multiple paths. Each step, or “thought,” is represented within the tree, and the model evaluates the viability of each step. Ineffective thoughts are abandoned, and the model continues exploring other branches until it reaches the desired result.
How is ToT prompting visually represented in illustrations?
ToT prompting is depicted with rectangular boxes representing individual thoughts within the reasoning process, forming a branching structure. In contrast, the CoT prompting illustration shows a more linear thought process.
What were the results of tests conducted with ToT prompting, and in what context was it found to be effective?
Tests, including a mathematical card game called “Game of 24,” showed that ToT prompting consistently outperformed other approaches. However, it was noted that ToT prompting might not be necessary for tasks that language models like GPT-4 already handle well.
Featured Image Credit: Photo by Mojahid Mottakin; Unsplash – Thank you!
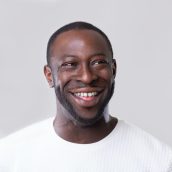
Colin Hughes, a passionate wordsmith and digital raconteur. He ghostwrites for numerous websites that include travel, culture, and lifestyle content. When not traveling for work, he loves to spend his time at home with his husband and two border collies, Reggie and Tuesday.