The Future of Algorithmic Evaluation: Machine-Led Relevance Labeling


The landscape of algorithmic evaluation in search engines is undergoing a profound transformation. Recent research by Bing and a surge in related information retrieval studies suggest that significant changes are on the horizon. These changes have the potential to revolutionize the role of human quality raters and the frequency of algorithmic updates. By leveraging large language models (LLMs) and machine-led relevance labeling, search engines can overcome the scalability issues and limitations of human evaluators. This article explores the implications of this shift and the possibilities it presents for the future of algorithmic evaluation.
The Importance of Evaluation in Search Engines
Evaluation is a critical step in the search engine process, alongside crawling, indexing, ranking, and result serving. It involves assessing the alignment between search results and the subjective notion of relevance to a given query, at a specific time and within a specific user context. As query relevance and user information needs evolve, search result pages must also adapt to meet the intent and preferred user interface of search engine users. These changes can be predictable, such as temporal shifts in query intent during seasonal events, or they can be more nuanced and unpredictable.
To ensure that proposed changes in rankings or experimental designs are truly better and more precise in addressing user information needs, search engines rely on evaluation. This stage involves the input of humans who provide feedback and assessments before the changes are implemented in production environments. While evaluation is an ongoing part of production search, it is crucial during the development phase to validate the effectiveness of proposed changes and gather substantial data for further adjustments if necessary.
Human-in-the-Loop (HITL) Evaluation
Data labeling is an integral part of the evaluation process. It involves marking data to transform it into a format that can be measured at scale. Crowd-sourced human quality raters have traditionally been the primary source of explicit feedback for search engine evaluation. These raters, hired through external contractors and trained with detailed guidelines, provide synthetic relevance labels that emulate real search engine users. Their feedback often takes the form of pairwise comparisons of proposed changes to existing systems or other proposed changes.
However, the use of the crowd for relevance labeling poses challenges. Scalability is a significant issue, with the demand for data labeling growing exponentially across various industries. Moreover, human evaluators are prone to errors, biases, and other cognitive factors that can affect the quality and consistency of their relevance assessments. The reliance on human evaluators also raises ethical concerns, particularly regarding the low pay and working conditions of crowd workers in emerging economies.
Implicit and Explicit Evaluation Feedback
Search engines employ both implicit and explicit feedback for evaluation purposes. Implicit feedback refers to user actions that indicate relevance or satisfaction without their active awareness. This includes click data, user scrolling behavior, dwell time, and result skipping. Implicit feedback is valuable for understanding user behavior and informing learning-to-rank machine learning models. However, it comes with inherent biases and challenges in determining the true intent behind user actions.
Explicit feedback, on the other hand, involves actively soliciting user judgments on search results’ relevance or helpfulness. Search engines may directly ask users for feedback or rely on user research teams to collect relevance data labels for specific queries and intents. These data labels are then used to adjust and fine-tune proposed ranking algorithms or evaluate experimental designs. While explicit feedback provides more precise relevance assessments, it requires significant resources and is not easily scalable.
The Challenges of Crowdsourcing Relevance Labeling
Crowd-sourced human quality raters have been the backbone of relevance labeling for search engines, replacing professional expert annotators as the need for scalability grew. However, the use of the crowd presents several challenges. Task-switching among different evaluation tasks can lead to a decline in the quality of relevance labeling. There is also evidence of left-side bias, where results displayed on the left side of a comparison are more likely to be perceived as relevant. Anchoring, whereby the first relevance label assigned by a rater influences subsequent labels, can also impact the accuracy of evaluations.
General fatigue among crowd workers and disagreements between judges on relevance labeling further complicate the process. Moreover, the reliance on low-paid crowd workers in data labeling raises ethical concerns and highlights the need for a more scalable and reliable alternative.
The Rise of Machine-Led Evaluation
To address the scalability and quality limitations of human evaluators, search engines are exploring the use of large language models (LLMs) as machine-led relevance labelers. Bing, in particular, has made significant progress in this area, leveraging GPT-4 for relevance judgments. This breakthrough allows for the generation of bulk “gold label” annotations at a fraction of the cost of traditional approaches.
The use of LLMs as relevance labelers offers several advantages. It eliminates the scalability bottleneck associated with human evaluators and reduces costs. Machine-led evaluation also provides the potential for higher-quality relevance assessments, as LLMs can emulate the judgments of human quality raters. Bing’s research shows that models trained with LLMs can produce better labels than third-party workers, leading to notably improved rankers.
The Feasibility of Machine-Led Evaluation
The feasibility of machine-led evaluation is a topic of ongoing research and discussion in the information retrieval field. While there are concerns about the potential degradation of annotation quality with a complete shift towards machines, researchers are exploring different approaches to strike a balance between human and machine collaboration. A spectrum of human-machine collaboration, ranging from human-assisted annotations to machine-generated judgments, is being explored to optimize evaluation processes.
The future of algorithmic evaluation may involve a hybrid approach that leverages the strengths of both humans and machines. Human relevance assessors may provide more nuanced query annotations to assist machines in evaluation or act as supervisors and examiners of machine-generated annotations. This spectrum of collaboration allows for flexibility and adapts to the evolving needs of search engines and users.
Implications for the Future of Algorithmic Evaluation
If search engines embrace machine-led evaluation processes, significant changes can be expected in the frequency and agility of algorithmic updates. The scalability and cost-efficiency of machine-led evaluation enable more frequent updates and adjustments to meet evolving user information needs. Algorithmic updates can become more iterative and targeted, reducing the disruptive impacts of broad core updates.
The use of LLMs and machine-led evaluation also opens up possibilities for expanding evaluation efforts in non-English languages and specialized domains. By generating synthetic data and leveraging machine learning, search engines can overcome the limitations of traditional evaluation methods and offer more precise and relevant search results to users worldwide.
While challenges and risks exist, search engines must navigate responsibly and address potential biases and ethical concerns. Close monitoring and ongoing research are essential to ensure the reliability and fairness of machine-led evaluation processes.
In conclusion, the future of algorithmic evaluation lies in the integration of machine-led relevance labeling. By leveraging large language models, search engines can overcome scalability issues, reduce costs, and improve the quality of relevance assessments. The shift towards machine-led evaluation processes holds great potential for more frequent updates, targeted improvements, and enhanced user satisfaction in the ever-evolving digital landscape.
See first source: Search Engine Land
FAQ
Q1: What is the importance of evaluation in search engines?
A1: Evaluation is a crucial step in the search engine process that involves assessing the alignment between search results and user information needs. It ensures that proposed changes or experimental designs meet user intent and interface preferences. Evaluation is essential for validating changes and gathering data for further adjustments.
Q2: What is Human-in-the-Loop (HITL) evaluation?
A2: HITL evaluation involves using human quality raters to provide relevance feedback for search engine evaluation. These raters assess and provide feedback on proposed changes or experimental designs, emulating real user relevance judgments.
Q3: What are the challenges of using human quality raters for relevance labeling?
A3: Challenges of using human quality raters include scalability issues, potential errors, biases, cognitive factors affecting assessments, and ethical concerns regarding low pay and working conditions of crowd workers.
Q4: What are implicit and explicit feedback in search engine evaluation?
A4: Implicit feedback includes user actions that indicate relevance or satisfaction without their active awareness, such as clicks and dwell time. Explicit feedback involves actively soliciting user judgments on search results’ relevance or helpfulness through direct user queries or user research teams.
Q5: What challenges does crowdsourcing relevance labeling present?
A5: Crowdsourcing relevance labeling challenges include task-switching, left-side bias, anchoring, general fatigue among crowd workers, and disagreements between judges on relevance labeling.
Q6: How are large language models (LLMs) being used in machine-led evaluation?
A6: LLMs like GPT-4 are used for machine-led relevance labeling in search engine evaluation. They generate “gold label” annotations at a lower cost compared to traditional approaches, offering the potential for higher-quality relevance assessments.
Q7: What is the feasibility of machine-led evaluation?
A7: The feasibility of machine-led evaluation is being researched, with a focus on striking a balance between human and machine collaboration. A spectrum of collaboration approaches, from human-assisted annotations to machine-generated judgments, is explored to optimize evaluation processes.
Q8: What are the implications of machine-led evaluation for the future of algorithmic updates?
A8: Machine-led evaluation can lead to more frequent and agile algorithmic updates. It reduces the scalability bottleneck and enables iterative and targeted updates to meet evolving user information needs. Algorithmic updates can become less disruptive with this approach.
Q9: How can machine-led evaluation expand evaluation efforts?
A9: Machine-led evaluation enables search engines to expand evaluation efforts in non-English languages and specialized domains. It overcomes the limitations of traditional methods, offering more precise and relevant search results worldwide.
Q10: What challenges and risks should search engines consider with machine-led evaluation?
A10: Search engines should address potential biases, ethical concerns, and the reliability and fairness of machine-led evaluation processes. Ongoing research and monitoring are essential to ensure responsible and ethical use of this technology.
Featured Image Credit: Photo by Myriam Jessier; Unsplash – Thank you!
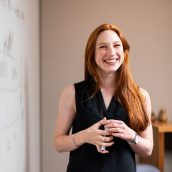
Olivia is the Editor in Chief of Blog Herald.